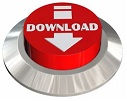
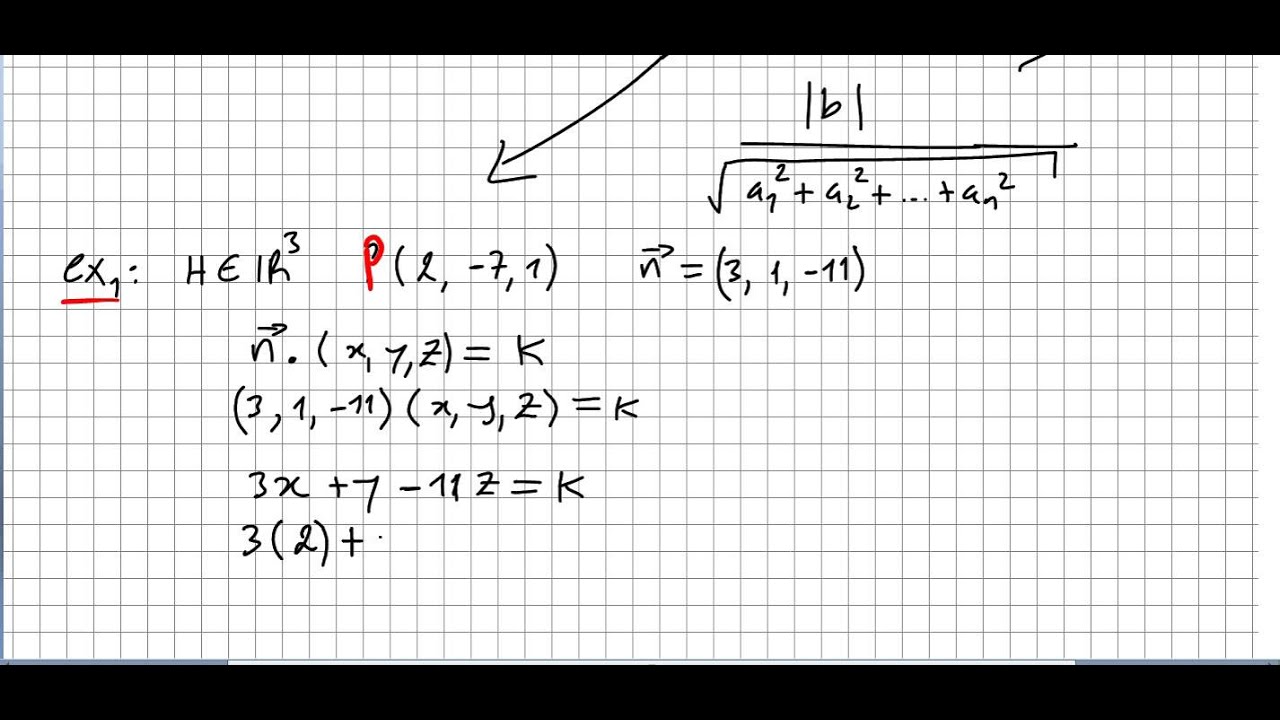
The decision boundary is the set of points of that hyperplane that pass through 0 (or, the points where the score is 0), which is going to be a hyperplane with K-1 dimensions. However, our views differ in an important. SVM is not scale invariant, so it’s highly recommended to scale your data. In general, there is a hyperplane of K dimensions that defines the score of the classifier. (sets of pairs of a world and norm), or Gibbard s fact-plan contents (sets of pairs of a world and hyperplan).If you have categorical inputs, you may need to covert them to binary dummy variables (one variable for each category). Numerical Inputs: SVM assumes that your inputs are numeric.It doesn’t perform very well when the dataset has more noise, i.e.SVMs do not directly provide probability estimates-these are calculated using an expensive five-fold cross-validation process (see scores and probabilities, below).The same set of parameters will not work optimally for all use cases. The main disadvantage of SVM is that it has several key parameters like C, kernel function, and Gamma that all need to be set correctly to achieve the best classification results for any given problem.Its primary objective is to precisely define global units (systems, series, and stages) of the International Chronostratigraphic Chart that, in turn, are the basis for the units (periods. It works really well with a clear margin of separation. The International Commission on Stratigraphy is the largest and oldest constituent scientific body in the International Union of Geological Sciences (IUGS).Realists consider that moral claims do report facts and that their truth. Common kernels are provided, but it’s also possible to specify custom kernels. A reconsidered version of Gibbards hyperplan could be used to solve the problem. labeling of norms as hyperplans, in Gibbard 2003, doesnt affect. SVM is versatile: different kernel functions can be specified for the decision function. ence between his view and Gibbards is rather slight.It’s still effective in cases where the number of dimensions is greater than the number of samples.It’s very effective in high-dimensional spaces as compared to algorithms such as k-nearest neighbors.However, by using a nonlinear kernel as mentioned in the scikit-learn library, we can get a nonlinear classifier without transforming the data or doing heavy computations at all. Normally, the kernel is linear, and we get a linear classifier. What especially distinguishes the quasi-realist project is an emphasis on explaining why we are entitled to act as if moral judgments are genuinely truth-apt even while strictly speaking they are. Different kernel functions applied on Iris-Dataset Yet other sophisticated non-cognitivists, notably Allan Gibbard, have been happy to work under the quasi-realist banner (Gibbard 2003, 1819).
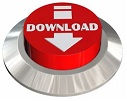